Human Factors in Recommender Systems in Requirements Engineering
My dissertation research is focused on Human Factors in Recommender Systems in Requirements Engineering (RE). Several studies have applied recommender technologies to support requirements engineering activities. While many studies have focused on the algorithms’ prediction accuracy, there have been fewer discussions around users’ in RE it is important to also explore the systems from the user's perspective.
Current Study
Beyond the Algorithm: User Evaluation of Recommender Systems in Requirement Elicitation
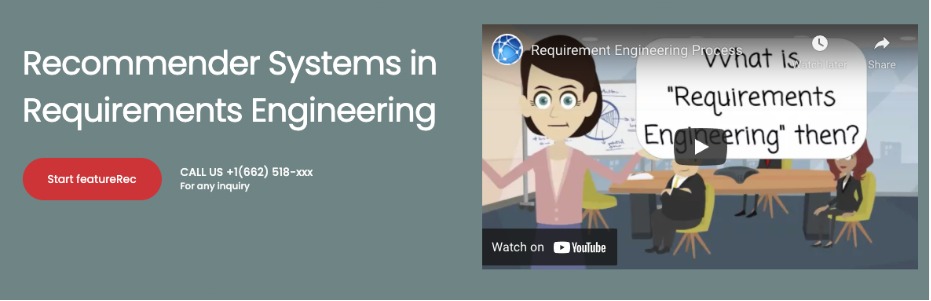
This work explores RE recommender systems from a human factors perspective. It investigates the impact system-generated recommendations have on users’ decision processes in the requirement elicitation activity. Existing works on RE recommenders have been based on offline experiments mainly focused on the prediction accuracy of the underlying algorithms. However, high prediction accuracy does not necessarily correlate with high adoption of the recommendations.
To what extent higher accuracy translates into higher efficiency for the user, improved user satisfaction, or acceptance of the service has not been fully clear. To that end, in this work, we present our research questions and perform a preliminary user study using a recommender - ‘featureRec’ that we designed for this purpose. We aim to understand to what extent users actually rely on such a recommendation service in the first place and how they influence users’ decision-making behaviors and outcomes.
View screenshots of featureRec